The Burr II-R{Y} Family of Distributions
Clement Boateng Ampadu*
Department of Biostatistics, USA
Submission: October 24, 2019; Published: November 20, 2019
*Corresponding author: Clement Boateng Ampadu, Department of Biostatistics, USA
How to cite this article: Clement Boateng Ampadu. The Burr II-R{Y} Family of Distributions. JOJ Wildl Biodivers. 2019: 1(5): 555575 . DOI: 10.19080/JOJWB.2019.01.555575
Abstract
The Burr system of distributions [1] arise from a differential equation with solution
where g(x) is a function whose integrals are such that F(x) increases from 0 to 1 on the interval −∞ < x < ∞. Inspired by the T − R {Y} framework of creating probability distributions [2], this paper assumes T is a Burr II random variable, to introduce also-called Burr II-R{Y} family of distributions. A member of this family is shown to be a good fit to the precipitation data [3]. Finally, as this article is introductory in nature, the reader is asked to further investigate some properties and applications of this new class of statistical distributions.
Keywords: T-R{Y} family of distributions; Burr system of distributions; Precipitation data
Contents
a) Introduction and the New Family
b) Practical Illustration
c) Concluding Remarks and Further Recommendations
Introduction and the new family
Let T, R, Y be random variables with CDF’s
On the other hand, the CDF of the Burr II distribution is given
where < x<,−∞∞By differentiation, the PDF of the Burr II distribution is given by
From the CDF of the T − R {Y} family of distributions we have the following
Proposition 1.1. The CDF of the Burr II-R{Y} family of distributions is given by
where the random variable Y has quantile QY , r > 0, and the random variable R has CDF FR. The parameter space of ξ and x depends on the chosen baseline distribution of the random variable R.by differentiating the CDF in the previous Proposition, we have the following.
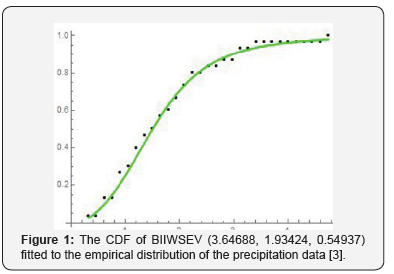
Proposition 1.2. The PDF of the Burr II-R{Y} family of distributions is given by
Practical illustration
We assume R is a Weibull random variable with the following CDF
for x, a, b > 0. We assume Y is standard extreme value, so that
for 0 < p < 1. Now from Proposition 1.1, we have the following
Corollary 2.1. The CDF of the Burr II-Weibull {Standard Extreme Value} distribution is given by
where x, a, b, r > 0
Notation 2.2. We write c BIIWSEV (a, b, r), if C is a Bur IIWeibull {Standard Extreme Value} random variable.
Concluding Remarks and Further Recommendations
In this paper we introduced a so-called Burr II-R{Y} family of distributions and showed a member of this class of distributions is a good fit to the precipitation data [3]. As this paper is introductory in nature; we ask the reader to further explore some properties and applications of this new class of distributions.
References
- Burr IW (1942) Cumulative frequency functions. Annals of Mathematical Statistics 13: 215-232.
- Aljarrah (2014) On generating T-X family of distributions using quantile functions Journal of Statistical Distributions and Applications 1: 2.
- Suleman Nasiru, Peter N, Mwita, Oscar Ngesa (2018) Discussion on Generalized Modified Inverse Rayleigh, Appl Math Inf Sci 12(1): 113-124.
- Ayman Alzaatreh, Carl Lee, Felix Famoye (2014) T-normal family of distributions: a new approach to generalize the normal distribution, Journal of Statistical Distributions and Applications 1: 16.