Performance of Soilwat Model for Soil Physical Properties Simulation in Auchi, Edo State
Ajayi AS1*, Eriakha EC1, Abu Malik2 and Ibrahim Rasheed1
1Department of Agricultural and Bio-Environmental Engineering Technology, Auchi Polytechnic, Nigeria
2Department of Agricultural Technology, Auchi Polytechnic, Nigeria
Submission: December 04, 2018; Published: January 17, 2019
*Corresponding author: Ajayi AS, Department of Agricultural and Bio-Environmental Engineering Technology, Auchi Polytechnic Auchi, Edo State Nigeria
How to cite this article: Ajayi A, Eriakha E, A Malik, Ibrahim R. Performance of Soilwat Model for Soil Physical Properties Simulation in Auchi, Edo States. Int J Environ Sci Nat Res. 2019; 19(1): 556005. DOI:10.19080/IJESNR.2019.19.556005
Abstract
The physical properties of soils are dominant factors affecting the use of a soil which determine the availability of O2 in soils, the mobility of water into and though soils and case of root penetration and the chemical and biological behavior of soil. The aim of this paper was to investigate the performance of soil physical properties data was OILWAT model in the prediction of soil physical properties. adapted from previous study in the Department of Agricultural and Bio-Environmental Engineering Technology. SOILWAT performed poorly in simulating volumetric moisture content, the equation of the line obtained is y = 0.0403x + 15.428, with the R² = 0.0274, the RMSE value is 4.21 which is quite large compare to the model’s ability to simulate bulk density and saturated hydraulic conductivity. When compared with the measured data, SOILWAT significantly simulated Bulk Density (BD) for Sandy loam and sandy clay loam soil having a perfect agreement between the observed and simulated values (R2 = 0.90, p<0.05; RMSE of 0.033 and regression equation given as; y = x – 0.33). The model significantly predicted the Saturated hydraulic conductivity, with R2 value of 0.92 and root mean square error (RMSE) 0.03, the equation of best fit obtained is y = x+0.21, since sample environment (confinement and overburden) are not represented in laboratory procedures, laboratory data may not always agree with field data.
Keywords: Soil; Mobility; Biological behavior, Inorganic particles; Agro-ecological; Agro-hydrological
Introduction
The physical properties of soils are dominant factors affecting the use of a soil which determine the availability of O2 in soils, the mobility of water into and though soils and case of root penetration and the chemical and biological behavior of soil. These depend primarily on the amount, size, shape and arrangement of its inorganic particles, shape and arrangement of its inorganic particles, kind and amount of organic matter, the total volume of pore spaces and the way it is occupied by water and air at a time [1].
The need to know the physical condition of the soil occurs frequently in many agro-ecological and agro-hydrological investigations. This is because soil physical properties play a vital role in plant water storage, utilization, plant growth and development. In irrigated agriculture for instance, information on local soil properties is required for water budgeting for irrigation planning as well as the actual scheduling of irrigation. The chances of over- or under- irrigation is high in the absence of information about the soil moisture content status in the soil profile. In hydrology, knowledge of the degree of soil wetness is useful in the estimation of runoff, sediment yield of catchment and flood discharge. Knowledge of soil moisture status is also equally important in geo-hydrological investigations and in design of soil erosion control measures.
As a result of the importance of knowing soil physical properties, so many methods and devices have evolved over the years to either directly measure or estimate soil moisture content. Measurement and analyses of the soil physical properties are difficult, costly and time consuming. Hence, the use of expensive special equipment becomes necessary. Several research studies over last three decades has formulated models, which enables its determination based on measured soil physical and chemical properties, which serves as inputs [2-4].
These models are referred to as pedotransfer functions (PTFs) [5]. Soil physical property modelling is defined as the dynamic simulation of hydrologic processes by numerical integration of individual processes with the aid of computer [6]. A better understanding of agricultural soil management and hydrological analyses to a form a reliable predictive soil characteristics system will be dependent upon simulation modelling [4].
The Soil and Water Assessment tool (SOILWAT) is a modelling software package to analyse water, soil, agriculture and nutrient interactions at catchment modelling. This is a technology used to construct a relatively transparent surrogate (substitute) for the real soil water, then combined into a more comprehensive results and analysed by statistics which can be manipulated with far greater ease than the complex original [4].
A soil-water (SOILWAT) model capable of simulating soil hydrological properties of soil texture will help scientist in providing crucial data set for better understanding of our soils for better management. These data are also critical requirements in crop simulation models for decision making aimed at obtaining optimum results. Xue et al. [7] compared soil moisture observations with modeling results, he reported that the soil hydraulic parameters have a profound impact on the model simulations. The objective of this study was to compare the prediction made by SOILWAT model with the measured soil parameters and evaluate the general applicability and prediction accuracy of SOILWAT model for the predominant soil types in the derived savannah of Southwestern Nigeria.
The objective of this study is the performance of selected physical properties simulation models in Auchi, Edo State. To achieve this objective, the following specific objectives will be carried out: To measure/collect basic soil physical properties of the selected site. To simulate soil physical properties SOILWAT. To carry out comparative analysis to check the ability of the model to predict soil properties using statistical tools.
Adequate water resource management is essential for stable and efficient crop production especially under irrigated agriculture. Hence, efforts are being directed towards water management and conservation activities such as irrigation and control of flood and erosion. Realistic planning of these water management activities requires enough information on physical properties [8]. Data on rates of infiltration of water into soils can be used to supplement other soil information which could assist soil scientists, engineers, hydrologists and others to deal more effectively with a wide spectrum of water resource management and conservation problems.
The result of this study will provide researchers and scientists information on soil physical properties simulation models and their adaptability to field and laboratory studies.
Materials and Methods
Description of the study area
Auchi is located between latitude 60 70 ”00” to 70 18 ”00” North of the Equator and longitude 60 49 ”00” East of the Greenwich Meridian. Auchi is the headquarters of Etsako-West Local Government Area of Edo State, Nigeria. The area covers a total land area of 94,562km2. The area is found in the South Central (lower Niger sedimentary rock areas). The sedimentary rocks in the area are easily broken down by the incidence of water which results in the removal of particles of the rock. Auchi area is generally sloppy and as a result, the topography allows the easy movement of soil particles such that with little rainfall, rapid movement of materials is encouraged. The soil also contains abundant clay particles. Since clay particles contain little air, they readily hold water which forms a tenacious, sticky mass. Since percolation of surface water is reduced to the bearest minimum by the considerable low absorptive capacity of the sandy-clay particles, the heavy rainfall in the study area on a relatively undulating sloppy terrain acts therefore as a catalyst by making the soil easily saturated and erodible.
Soil physical properties data
Soil particle (sand, silt and clay) data was obtained from previous studies in the study area. Twenty set of data as seen in Table 1 was previously determined by Victory et al. [9] in the Department of Agricultural and Bio-Environmental Engineering Technology, Auchi Polytechnic Auchi. This data served as the basic data for estimating soil physical properties parameters using the model.
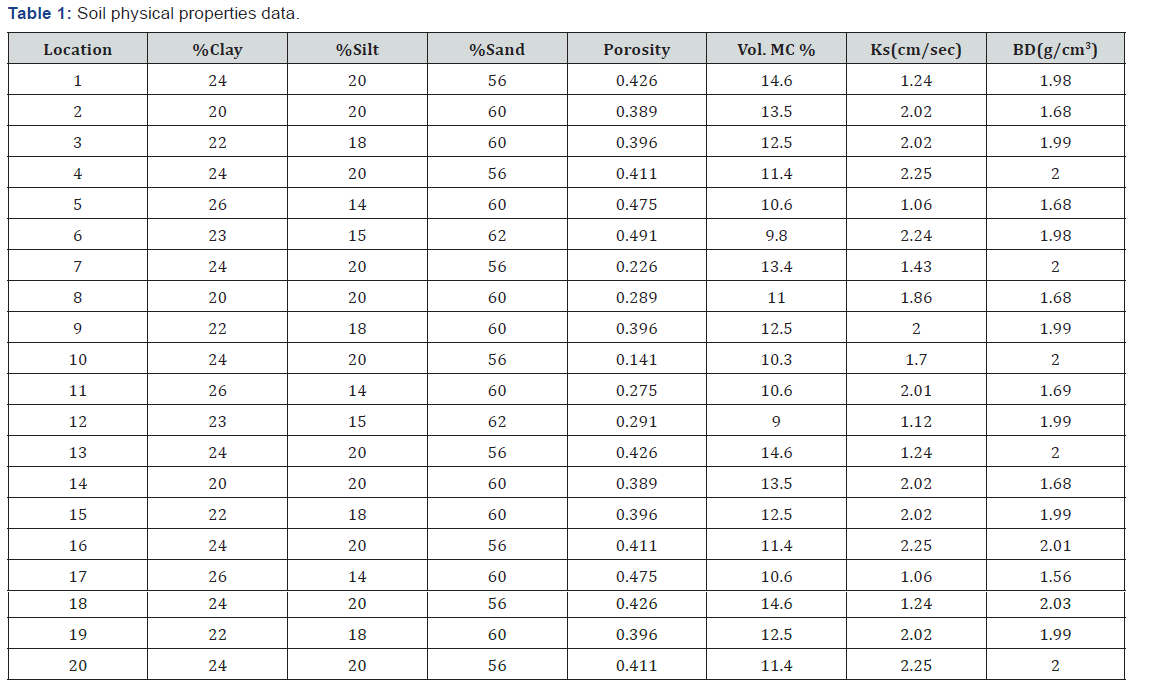
Source: Victory et al. (2016).
Estimation of model parameters
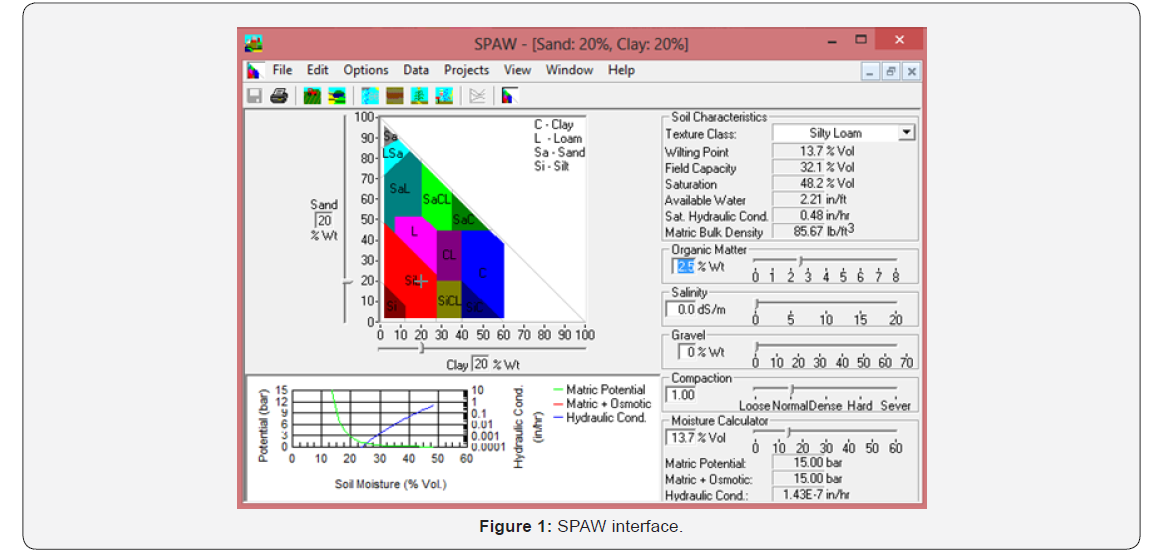
In order to assess the performance of the selected models in predicting soil physical properties, for both models studied the basic parameter for evaluation was the soil particle data (sand, silt and clay content).
Particle size analyses of the soils adapted from Victory et al. [9] indicates a sand, loamy sand, sandy loam and sandy clay loam textural classes. This variation in textures was used as the basis for grouping the soils and subsequently, for easy computation of the data sets for verification by the model; since the texture predominately determines the water holding characteristics.
The sand, silt and clay properties inputted into the model gives the values of other soil physical properties as seen in Figure 1.
Statistical analysis
For comparison of the difference between predicted soil water characteristic parameters and observed values, coefficient of determination (R2) as a goodness-of-fit index of agreement and the Root Mean Square Error (RMSE) were computed. Willmott [10] described RMSE as ‘among the best overall measures of model performance’, of which RMSE is more sensitive to extreme values due to its exponentiation; it therefore can be considered as a high estimate of the actual average error. The index of agreement is a standardized measure (scale 0-1) of the degree to which a model’s predictions are error free. yi denotes the measured value, i y -the predicted value, Y the average of the measured value and N is the total number of observations:
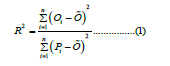
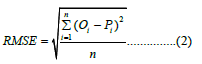
Where: Pi = predicted values, Õ = mean of the observed data, Oi = observed values, n = number of samples.
Results and Discussion
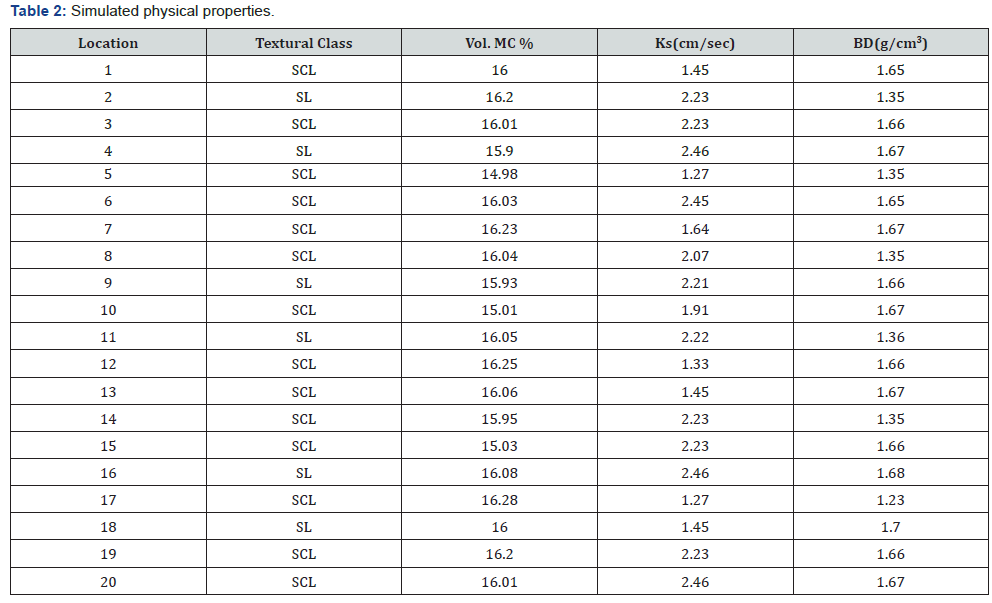
*SCL = Sandy clay loam, SL = Sandy loam, Ks = saturated hydraulic conductivity, BD = Bulk density, Vol MC = Volumetric moisture content.
The result obtained from model estimated soil physical properties using particle size data presented in Table 1 is shown in Table 2.
Comparison of observed and simulated soil physical properties
The values of the parameters estimated shown in Table 1 above were incorporated into the model to simulate physical properties of the soil. Table 2 & 3 shows the observed and simulated physical properties with their respective R2 and RMSE values in the goodness of fit chart shown in Figure 2-4.
Discussion of Results
From Table 3 and Figure 2 it can be seen that SOILWAT performed poorly in simulating volumetric moisture content, the equation of the line obtained is y = 0.0403x + 15.428, with the R² = 0.0274, the RMSE value is 4.21 which is quite large compare to the model’s ability to simulate bulk density and saturated hydraulic conductivity.
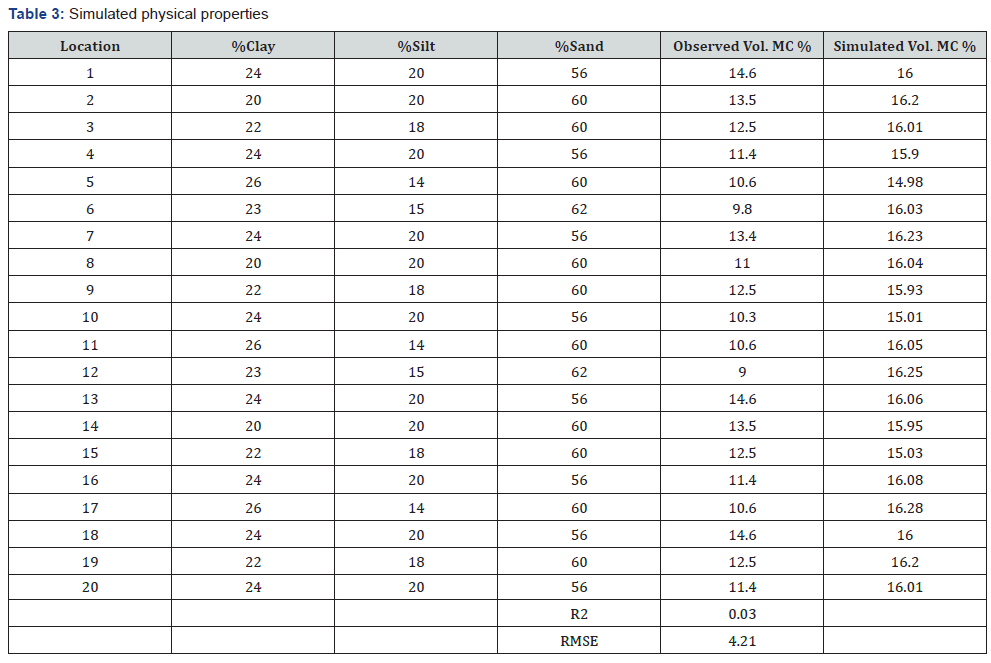
*SCL = Sandy clay loam, SL = Sandy loam, Ks = saturated hydraulic conductivity, BD = Bulk density, Vol MC = Volumetric moisture content.
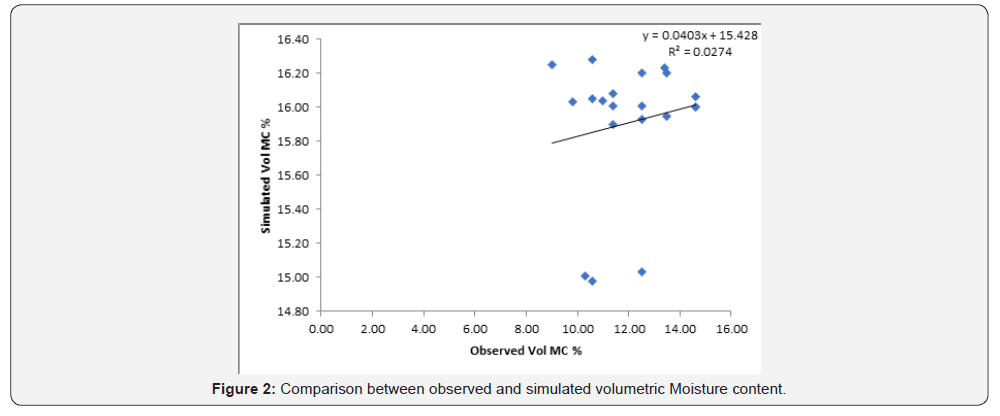
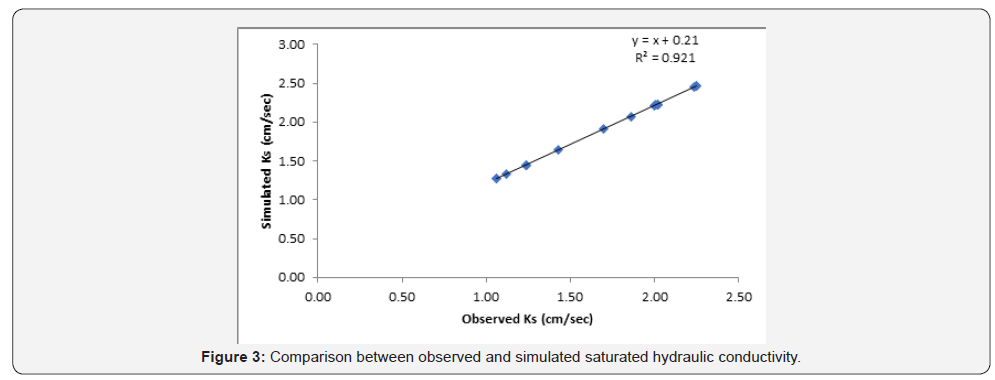
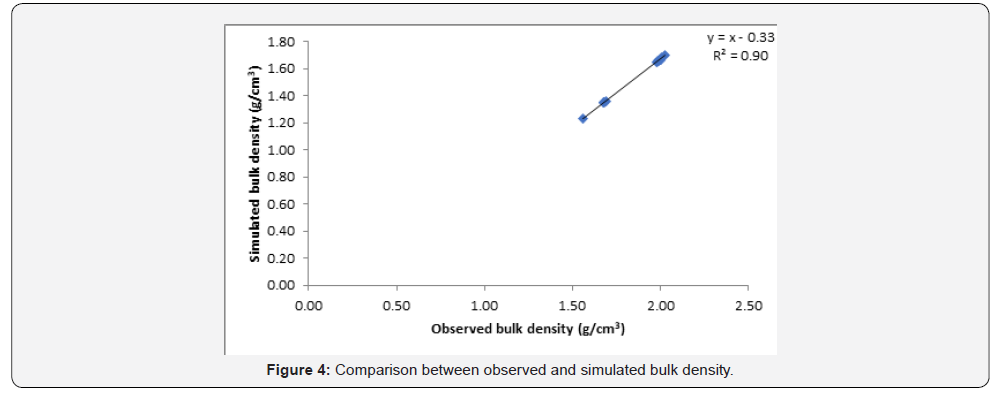
Bulk density: When compared with the measured data, SOILWAT significantly simulated Bulk Density (BD) for Sandy loam and sandy clay loam soil having a perfect agreement between the observed and simulated values (R2 = 0.92, p<0.05; RMSE of 0.033 and regression equation given as; y = x – 0.33) as presented in Table 4 & 5 and Figure 4. The relationship between the simulated and measured values showed that there is no fluctuation in the measured values simulate by the model; which indicated a good prediction. The concentration of the sand soil in one site i.e., non-uniformity of the distribution of sand texture class amongst the sites may be one among other factors responsible for the little error detected by the RMSE indices. Gijsman AJ et al. [11] reported that the SOILWAT, though, performed best among other models compared in his studies, but this does not apply to all soils. For very sandy soils, no method performed well. The high number of coarse-size particles in the sandy soils is possibly the reason for the fairly good-fit by the model [12].
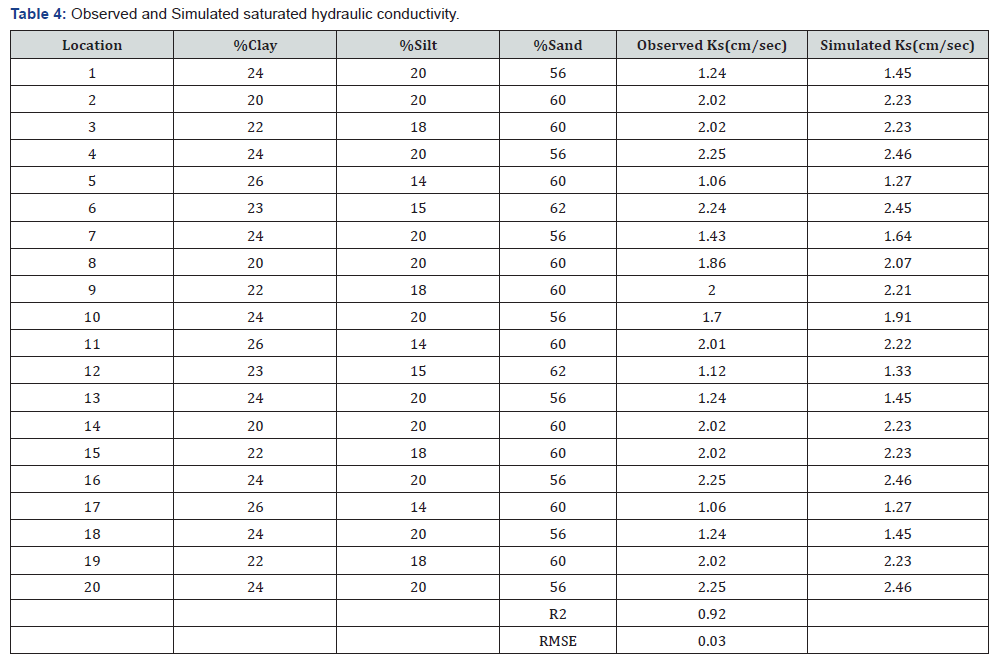
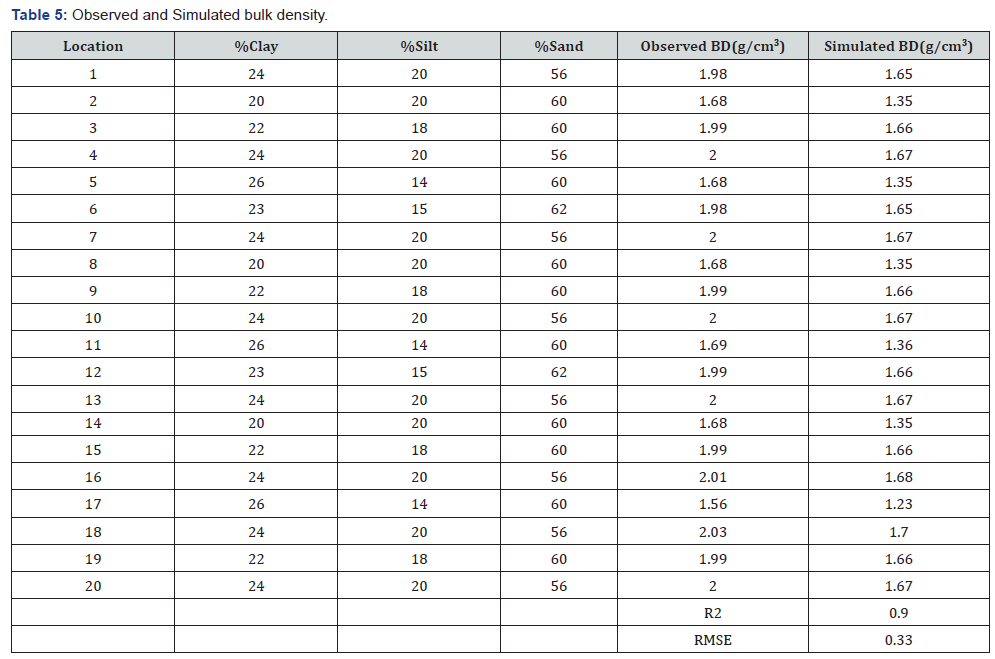
SOILWAT significantly predicted the Saturated hydraulic conductivity, with R2 value of 0.90 and root mean square error (RMSE) 0.03, the equation of best fit obtained is y = x+0.21, since sample environment (confinement and overburden) are not represented in laboratory procedures, laboratory data may not always agree with field data. The disagreement appears more pronounced at high water contents [13-16].
Conclusion
The SOILWAT model resulted in higher coefficient of determination for Sandy loam soil which expresses the goodness-of-fit between the simulated and measured values. However, the poorfit measurement of the model for sand, loamy sand and sandy clay loam might be as a result of the sensitivity of the model in terms of location or site-specific and the high gravel content of the sampled soils which made up this textural class. The SOILWAT model has a tolerable range of 0-60%. The ability of SOILWAT to simulate soil water characteristics for sandy loam soil demonstrates the potential of the model when properly initialized and field measurement accurately taken. SOILWAT has shown the potential of serving as tool that would enable decision makers to explore the future of sustainable agriculture, even in developing countries where soil water extraction apparatus have become a limitation in determining soil water availability. Despite the optimistic position of system modelling, realization of the full potential depends considerably on availability and quality of inputs for running the model, taken into consideration location or site-specific information in developing the model.
Recommendations
Having achieved the objective of this study, it is therefore recommended that:
a) Other model types should be used to simulate soil physical properties for the study area.
More statistical tools should be used to check for further discrepancies between observed and simulated soil physical properties.
References
- Hijmans RJ, Forbes G, Walker TS (2000) Estimating the global severity of potato late blight with GIS-linked disease forecast models. Plant Pathol 49: 697-705.
- Rajkai K, Varallyay G (1989) Estimating soil water retention from simpler soil properties by regression techniques. Proceedings of the International Workshop on Indirect Methods for Estimating the Hydraulic Properties of Unsaturated Soils, Riverside, CA, USA.
- Williams RD, Ahuja LR, Naney JW (1992) Comparisons of methods to estimate soil water characteristics from soil particle size, bulk density and limited data. Soil Sci 153: 172-184.
- Saxton KE, Rawls WJ (2006) Soil water characteristics estimates by texture and organic matter for hydrologic solutions. Soil Sci Soc Am J 70: 1569-1578.
- Bouma J (1989) Using soil survey data for quantitative land evaluation. Adv Soil Sci 9: 177-213.
- Saxton KE, Rawls WJ, Romberger JS, Papendick RI (1986) Estimating generalized soil water characteristics from texture. Soil Sci Soc Am J 50: 1031-1036.
- Xue Y, Zeng FJ, Schossler CA (1996) SSiB and its sensitivity to soil properties- Acase study using HAPEX-Mobilhy data. Global Planet Change 13(1-4): 183-194.
- Ebaluana JI (2018) Performance of soil physical properties simulation models in auchi, edo state. Unpublished Higher National diploma project, submitted to the department of Agricultural and Bio-Environmental Engineering Technology, Auchi Polytechnic Auchi, Edo State.
- Victory Erazua, Friday A, Jovita Okhulegbe (2016) Construction and testing of single and double ring infiltrometer in Auchi Polytechnic Auchi. Unpublished National diploma project, submitted to the department of Agricultural and Bio-Environmental Engineering Technology, Auchi Polytechnic Auchi, Edo State.
- Willmott CJ (1981) On the validation of models. Phys Geogr 2: 184- 194.
- Gijsman AJ, Jagtap SS, Jones JW (2002) Wading through a swamp of complete confusion: How to choose a method for estimating soil water retention parameters for crop models. Eur J Agron 18(1-2): 77-106.
- Hwang SI, Powers S (2003) Using particle-size distribution models to estimate soil hydraulic properties. Soil Sci Soc Am J 67(4): 1103-1112.
- Arya LM, Dierolf TS (1992) Predicting soil moisture characteristics from particle-size distribution: An improved method to calculate pore radii. Proceedings of the International Workshop on Indirect Methods of Estimating the Hydraulic Properties of Unsaturated Soils, Riverside, CA, USA, pp. 115-124.
- FAO (1988) Soil Map of the World. Revised Legend, Reprinted with Corrections. World Soil Resources Report 60, FAO, Rome.
- Rawls WJ, Brakensiek DL, Saxton KE (1982) Estimating soil water properties. Trans Am Soc Agric Eng 25: 1316-1320, 1328.
- USDA/SCS (1982) Procedures for collecting soil samples and methods of analysis for soil survey. Soil Survey Investigations Report I, Washington, DC.