The Use of Texture Analysis on Transvaginal Ultrasound Images in Diagnosing Ovarian Masses: A Prospective Study
Aldahlawi Rana12* and Pugh Neil1
1Department of Medical Physics and Clinical Engineering, Cardiff University, UK
2Department of Radiological Sciences, King Saud University, Saudi Arabia
Submission: March 23, 2017; Published: April 25, 2017
*Corresponding author: Rana Hussain Aldahlawi, Department of Radiological Sciences College of Applied Medical Sciences, King Saud University, Riyadh, Saudi Arabia, Tel: +966 565232325; Email: rdahlawi@ksu.edu.sa
How to cite this article: Aldahlawi R, Pugh N. The Use of Texture Analysis on Transvaginal Ultrasound Images in Diagnosing Ovarian Masses: A Prospective Study. J Gynecol Women's Health. 2017; 4(2): 555632. DOI: 10.19080/JGWH.2017.04.555632
Abstract
Objective: To assess the diagnostic performance of texture analysis ^discriminating between benign and malignant adnexal masses
Design: prospective observational cross-sectional study
Population: Women aged eighteen and above with known pelvic masses
Methods: ultrasound images were collected from participants and transferred into PC for off-line analysis. MaZda software was used to perform the texture analysis. Two texture features applied: Grey-level co-occurrence matrix (GLCM) and wavelet. Risk of malignancy index,Pelvic mass index and ADNEX scoring systems were applied to the data, and then results were compared to GLCM and wavelet.
Main outcome measures: The GLCM showed a higher sensitivity (72%) compared to two of the scoring systems applied (32% RMI and 62% ADNEX). When combining GLCM and wavelet features using logistic regression model resulted in high performance with AUC=0.8 and a good predictive capacity when tested using the Hosmer and Lemeshow test (p=.502).
Results: 169 masses were collected, 116 benign, 29 malignant and 24 simple cyst. Data were divided into 95 premenopausal and 68 postmenopausal. There was a significance difference between benign and malignant p=.004, p=.027 for GLCM and wavelet respectively. GLCM had a higher sensitivity (72%) and NPV (90%) for the entire cohort in comparison to RMI (32%; 80%) and ADNEX (62%; 80%) respectively, Conclusions: Two features of texture analysis have a potential in differentiating benign from malignant pelvic masses which are (GLCM) and the wavelet.
Keywords: Ovarian masses; Texture analysis; GLCM; Pelvic ultrasound; RMI; ADNEX; PMI(pelvic mass index)
Abbreviations: GLCM: Grey-Level Co-Occurrence Matrix; RMI: Risk of Malignancy Index; PMI: Pelvic Mass Index; ADNEX: The Assessment of Different Neoplasias in the Adnexa; AUC: Area Under the Curve; UKCTOCS: United Kingdom Collaborative Trial of Ovarian Cancer Screening; CA-125: Cancer Antigens (Blood Test Marker for Cancer); IOTA: International Ovarian Tumor Analysis Group; ROI: Region of Interest; ROC: Receiver Operating Curve
Introduction
Ovarian cancer is the second most common gynecological malignancy; however, it remains the leading cause of death among these diseases [1]. Ultrasound is considered the main modality for ovarian cancer triage [2] however, a study in 2005 concluded that ultrasound has a high false-positive rate in the differential diagnosis of adnexal malignancies, even with several scoring systems [3].
Other workers have used Doppler to differentiate between benign and malignant ovarian masses to improve the specificity of ultrasound. Unfortunately, according to most of these studies, this approach does not add significant useful information, with a reported accuracy of only 35%- 88% [2,4].
Other preoperative methods to differentiate benign from malignant ovarian masses have been developed and tested recently, such as, 3D imaging [5] and using CT [6] or MRI imaging. However, they all have limitations, including limited availability, high cost, difficulty with the identification of small tumour deposits and the distinction between benign and pathological lesions [7,8].
The main issue is that the interpretation of the images is subjective and observer dependent [2]. Texture analysis has been shown to have potential in the objective assessment of ovarian cancer in a preliminary study [9].
In recent years, objective diagnostic methods have been proposed to overcome the subjectivity and operator dependence limitation [10]. However, there is no reliable technique available at present. Hence, a new objective method is desired to address the above mentioned issue which will contribute in patient management.
Texture analysis techniques have been successfully applied to various types of tissues and organs including the carotid artery [11], breast [12,13], heart [14], thyroid gland [15] and liver [16-18]. However, it has not yet been widely tested on ovarian tissue.
Texture analysis is a technique for evaluating the structure within an image. In digital imaging, texture analysis is the distribution of grey level values across the pixels of a given region of interest [19]. The variation in intensity reflects some physical variation in the underlying structure.
A preliminary study showed usefulness of texture analysis in differentiating ovarian lesions [9]. Therefore, objective differentiation between benign and malignant ovarian tissue through texture analysis on a prospective larger sample and compairing this new method to widely avaliable scoring systems would be beneficial by validating the accuracy of the method.
Study Aims
To assess prospectively the diagnostic performance of texture analysis on transvaginal ultrasound images in discriminating between benign and malignant adnexal tumors and to compare it to other widely used scoring systems.
Materials and Methods
This study is a quantitative prospective cross sectional study on women with known pelvic masses.This study took place in the Medical Physics and Clinical Engineering Department at the University Hospital of Wales (UHW), Cardiff, UK. A total of 226 patients fitted the criteria and were recruited for this study in the period between November 2013 and May 2015. Participants were selected from a pelvic mass clinic and Gynaecology oncology clinics. All patients with known adnexal mass were identified by members of the research team. The researcher approaches the potential participants and informed them of the research study
The study was explained and written information was provided. Patients were given up to 24 hours to decide if they wanted to participate in the study then an appointment was
booked for the scan. Written consent was taken before each scan.
The scanning procedure was the same in all participants in this study. All ultrasound procedures were undertaken by the researcher or the co-researchers only for the purpose of this study.
In women with bilateral ovarian masses, data from both sides were used for the analysis, i.e. complex morphology and simple cyst or dermoid on one side and a complex mass on the other one.
The following morphological ultrasound information was recorded in each case: volume of the ovary, site and volume of the cyst, cystic wall structure, and cystic wall thickness, presence of septation and septal thickness, presence of solid areas within the cyst, papillation height if present and echogenicity. As well as presence or absence of Doppler signal and the site of the signal were documented for each cyst.
These information were used to calculate three of the most commonly used scoring systems in ultrasound gynaecology clinics.
RMI risk of malignancy index
Originally developed by Jacobs and colleagues where (menopausal status, ultrasound findings and serum CA125) have been combined into diagnostic models: RMI=UxMxCA125 [20]. It has been evaluated in numerous primary studies. Each of the following grey-scale morphological features was given one point when present: bilateral lesions, multilocular lesions, solid areas, intra-abdominal metastases and ascites. If the sum of these points was 0, an ultrasound score U=0 was given, while a sum of 1 point an ultrasound score U=1, and a score of U=3 was given when the sum of ultrasound points ≥2 [20,21]. At a cut-off level of 200, the sensitivity is 85% and the specificity is 97% [22].
PMI (Pelvic mass index)
This scoring system combines transvaginal ultrasonography with Doppler; it is independent of CA-125. PMI assesses grey scale features such as size, laterality, presence of solid elements, septae and free fluid, all scoring one point each. The presence or absence of positive blood flow on Doppler ultrasound within the septa and/or solid component scores 2 points or -2 accordingly Peripheral blood flow within ovarian stroma is not considered significant. The maximum score is 7 and the minimum is -2. Scores between -2 and 0 are considered low risk, scores between 1 and 2 intermediate and scores of greater than 3 are associated with high risk of malignancy [23].
ADNEX scoring
The last scoring system is developed by the IOTA group, it is a new model called the ADNEX (the Assessment of Different NEoplasias in the adneXa). This model contains three clinical and six ultrasound predictors: age, serum CA-125 level, type of centre (oncology centres vs. other hospitals), maximum diameter of lesion, proportion of solid tissue, more than ten cyst locules, number of papillary projections, acoustic shadows, and ascites. This huge study was performed in twenty-four ultrasound centres in ten different countries with a total of 5,909 patients [24].
Their final ADNEX model is available online and in mobile applications
(www.iotagroup.org/adnexmodel/). This application has the advantage of calculating the risk even if the serum CA-125 level information is unavailable.
Materials
Toshiba Aplio 500 scanner with 6.0 MHZ Transvaginal transducer (model PVT-661VT) was used to acquire images. Standard presetting was used in all scans.
Transvaginal Ultrasound images were collected from participants and transformed to a PC as a BMP files for off-line analysis. MaZda software 4.6 (Institute of Electronics, University of Lodz, Poland) was used to perform the texture analysis. More details about this software are available online at: http://www. eletel.p.lodz.pl/mazda/. A ROI region of interest was drawn around the mass in each image then the two texture analysis features were selected (GLCM and Wavelet) then the sum of each features was documented.
GLCM is a second order statistical technique that allows for the extraction of statistical information from the image regarding the distribution of pair of pixels. It is computed by defining a direction, a distance and pair of pixels separated by this distance, computed across the defined direction [25].
Wavelet is a transform method of texture analysis. It is a tool that separates data into different frequency components. This feature measures the frequency content of the image on a given scale and in a given direction [26].
Histopathological diagnosis was obtained in women who underwent surgery and used as the gold standard. In cases where participants were managed conservatively and no histology results were available, ultrasound diagnosis by an expert examiner was used in typical adnexal masses (endometrioma, typical dermoid and simple cyst) as well as the use of a second diagnostic model such as MRI or CT where appropriate. Additionally follow-up at a minimum of 12 months after the ultrasound scan was used. Inclusion criteria: women age 18 and above with known pelvic masses. Exclusion criteria: women with other Gynaecological malignancy, i.e. not pelvic mass, pregnant patients, previous history of bilateral oophorectomy, difficult scans and unclear scan images and age less than 18 years old.
All data were statistically analysed using the Statistical Package Social Sciences (SPSS) program version 17.0 for windows (SPSS Inc, Chicago, Illinois, USA), The data in this study was non normaly distributed therefore, non parametric tests were used to calculate the median, SD such as Mann-Whitney test. Ninety-five percent confidence intervals were calculated where appropriate. The alpha level was set at 0.05 and any p-value less than the alpha level is considered statistically significant.
Results
163 patients (169 masses) were recruited for the purpose of this study. Ninety five were premenopausal and 68 postmenopausal. Their ages ranged from 18 to 53 in the premenopausal group (mean 41, median 42) and from 52 to 91 years (mean 67, median 66) in the postmenopausal group. Histopathology results of the masses showed 116 benign, 29 malignant and 24 simple cysts. Eighty one of the benign masses were premenopausal and 35 postmenopausal. Of the malignant masses 8 were premenopausal and 21 postmenopausal and of the simple cyst, 9 were premenopausal and 15 postmenopausal.
The values of the extracted features were compared in pairs: benign and malignant; cysts and malignant; and benign and cysts. The results for the GLCM and the wavelet features show that all group pairs were statistically significant: the p-value was <.05 in all groups.

The benign group was sub-divided into 4 groups which include: teratoma, endometrioma, fibroid and other suspicious or difficult to diagnose benign masses. The results of the comparison between these subgroups to the suspicious benign masses and to malignant masses are summarised in Table 1.
Receiver operating curve (ROC) analysis was performed to determine the ability of the GLCM feature in discriminating between cyst and benign masses, and between benign and malignant masses. An AUCclose to 1 indicates a strong discriminatory power/ability of the indicator variable while the AUC close to 0.5 indicates that the variable has little discriminatory power. A threshold value was selected to get the higher sensitivity possible with higher specificity possible. For instance, in discriminating between benign and malignant, the use of 245 as a threshold value led to an estimated sensitivity of 72% and specificity of 60%. Results are summaries in Table 2.
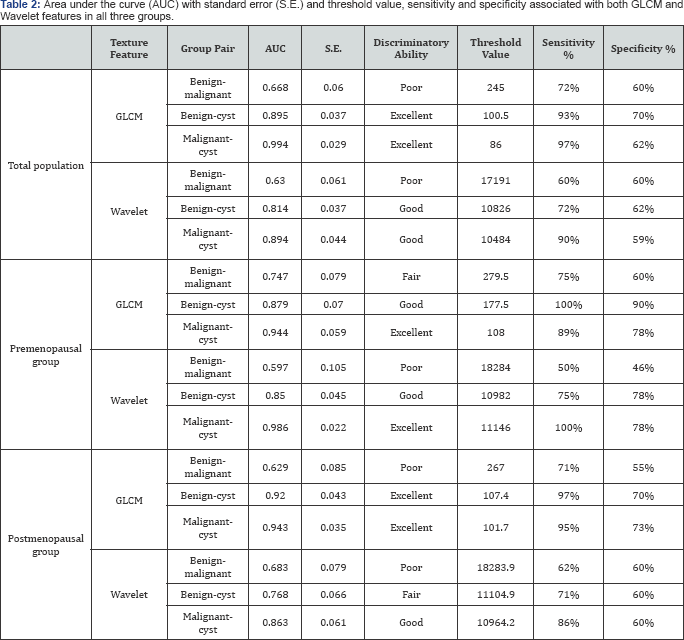
Further assessment was done using the widely used scoring systems which are: RMI, PMI, and ADNEX model. When applying RMI score on the data only 99 masses were applicable, 14 of them were excluded due to missing CA12 5 value;therefore, 85 masses were used in the analysis. One hundred and two of 169 masses were eligible for the PMI score. The results were divided into three groups, low risk (between -2- 0) intermediate (between 1-2) and high risk (above 3). The ADNEX model was applied on 81 of the 169 masses in this study. Results are shown in Table 3.

Subdividing the study population into the categories of pre- and postmenopausal status allowed more in-depth analysis of the performance of the three indices.
Ninety eight women were found in the premenopausal group; 81 benign, 8 malignant and 9 simple cysts. Similar to the total population analysis, group of pairs were compared to each other to test for significance. When using the GLCM all group pairs were still found to have a significance difference (p<0.05) in the premenopausal group. However when applying the wavelet feature, the malignant and benign masses could not be differentiated significantly (P=366). The other two group pair (benign- cyst and malignant-cyst) remained significantly different.
GLCM and PMI had the highest sensitivity (75%) compared to ADNEX and the wavelet (50%),and the lowest sensitivity was the RMI (14%). However, RMI had the highest specificity (95%) followed by the ADNEX (80%) and then the GLCM (60%) and the lowest was the wavelet (46%). As can be seen the sensitivity of wavelet,RMI, PMI and ADNEX (60,32,90 and 62% respectively in the total population) had dropped when analysing the premenopausal group specifically. While the performance improved in the GLCM (sensitivity was 72% in total population). In the specificity performance, RMI had improved from 87% to 95% as well as PMI from 51% to 77%, while it decreased in the wavelet from 60% to 48%. Moreover, no change is seen in both GLCM and ADNEX. Results are shown in Table 4.

Seventy one women were found in the postmenopausal group, 35 benign, 21 malignant and 15 simple cysts. Significance difference (p<0.05) was seen between the benign-cyst group pair and between the malignant and the cyst group pair. While in the difference between malignant and benign, no significance difference was found (p=110). All group pairs in the wavelet feature showed a significance difference, unlike the results from the premenopausal group where the difference between malignant and benign had no significance difference.
In this study, the ADNEX score was calculated for all masses whether CA125 results were available or not. However, as mentioned by Van Calster et al. [24] in their study where they develop the ADNEX model to differentiate between the different type of adnexal masses, it was found that CA125 was one of the strongest predictors and explained that deriving this model without the CA125 would decrease the discriminatory ability of the ADNEX. Therefore, it was decided to calculate the ADNEX score only for those who had the CA125 results so the difference could be appreciated.
It was found that out of the 81 eligible masses, 16 of them had a missing CA125, divided equally between benign and malignant. Moreover, when dividing the population by menopausal status, 6 were in the premenopausal group and 10 in the postmenopausal group. The ADNEX score was applied to 65 masses with available CA125 and resulted in 56% sensitivity, 81% specificity, 61% PPV, 77%NPV and 72% accuracy. Surprisingly, the sensitivity of the ADNEX model decreased slightly when using only masses with available CA125; for example in the total population the ADNEX sensitivity deceased from 62% to 56% and in the premenopausal group from 50% to 40% and lastly in the postmenopausal group from 66% to 62%. However, the specificity, PPV, NPV and the accuracy were similar to the ADNEX of all masses.
In order to improve the diagnostic performance, the two texture analysis features were combined [27]. Here the same threshold values were used together to assess the diagnostic performance of the GLCM and the wavelet combined. Therefore 245 was used as threshold value for the GLCM and 17191 for the wavelet simultaneously to indicate risk of malignancy. Results for the three groups are summarised in Table 5.

Further analysis was performed on the data using logistic regression to explore the relationship of the variables to the outcome (histology results or follow up). Testing for correlation between the variables was done to observe which of these variables are collinear,so that particular variable would not be used simultaneously in the equation. It was found that GLCM and wavelet are collinear and age and menopausal status as well. The final equation included menopausal status, wavelet and the ratio between wavelet and GLCM. This model showed to have good predictive capacity when tested using Hosmer and Lemeshow test (p=.502). Table 6 illustrates the results of the model. Moreover, Receiver operating curve (ROC) analysis was performed to determine the ability of this model to discriminate between benign and malignant masses and gave an AUC= 0.81 which has a good discriminatory ability.

Discussion
Ovarian cancer remains the leading cause of death in the Gynaecology malignancies. Up to this day, the nature of the mass has to be confirmed as malignant or benign by histology, which means having a surgical procedure.
Therefore, the use of accurate preoperative assessment of the risk of malignancy in adnexal masses is of high importance since the management of benign masses and ovarian cancer is quite different [28].
Main findings
Our data analysis showed that the sensitivity of the GLCM feature using a threshold value of 245 as indicative of malignancy was 72%, Our sensitivity of GLCM is considered low, the reason could be the fact that the appearance of many benign lesions overlaps with that of malignant disease [29].
These results are lower than some other published studies. For example, Xian [16] reported a sensitivity of 92% in a study where he applied GLCM texture feature to identify malignant and benign liver tumours on ultrasound images. This difference in sensitivity could be explained by the fact that texture analysis is more appropriate for the characterisation of regions exhibiting homogeneity in their structure as discussed by Diamond et al. [30].
The predictive value is an important measure for diagnostic test. Both positive and negative predictive values can be very important in the management and triage of patients with suspected ovarian cancer. For example, in the present study, a GLCM value of >279 in a postmenopausal woman gives a positive predictive value (PPV) of 47%. Although this probability is relatively low, it still limits unnecessary surgery to 2 women to identify one patient with ovarian cancer
This relatively low value could be explained by the low number of women diagnosed with ovarian cancer (29.6%) among our study postmenopausal group which is lower than previous studies that documented prevalence of 34% [16], but higher than PMI study which documented a prevalence of 16.7% [23]. Our results compares well with the UKCTOCS trial where they showed a low PPV of 5.3% and therefore, operating on 18.8 women for every cancer detected [31]. Although the UKCTOCS is a screening study which scans asymptomatic women which is different to our study where we scan known pelvic masses women, it still shows that our PPV is very good.
In the present study, a GLCM value of less than 245 had a NPV of 90% in the total population, which indicates that the probability that a woman with a GLCM value of less than 245 does not have ovarian malignancy is 90%. This findings is higher than 37.5% described by Zimmer et al. [32], close to previous PMI study NPV of 96% (23) and lower than 98% that been documented by Diamond et al. [16].
Our study showed that GLCM had better performance than both RMI and ADNEX model when differentiating benign from malignant masses, even when applying them to pre- and postmenopausal group separately. Although RMI should improve in performance when applied on the postmenopausal group, it still showed the lowest sensitivity (40%) among all the other scoring systems applied. Our results showed a much lower performance of RMI (ranges from 14-40%) when compared with previous studies that validate the RMI. For example the most recent systematic review and meta-analysis which investigate the diagnostic ability of several scoring systems calculated the pooled sensitivity and specificity of RMlas 72% and 92% respectively [33].
Although our results showed higher sensitivity of PMI (90%) compared to GLCM (75%), it has to be said that GLCM has the advantage of been objective over the subjectivity of the PMI score which is highly operator dependent.
The GLCM feature is the most commonly used in 2D texture analysis of medical images [34,35]. The results from this study demonstrated that in general, the GLCM has a better characterisation ability compared to wavelet feature, this is in accordance with the statement by Tuceryan [36]: the GLCM feature generally outperform other features. Likewise, in a study that focused on breast lesions [37] they reported that GLCM is the most useful feature and stated that GLCM has long been a powerful tool for texture analysis.
It was recommended in previous studies that combining texture features yields a better performance compared to using feature from a single category [27,38]. In our study we applied this theory using a logistic regression model, where it was found that applying wavelet with the ratio of wavelet to GLCM along with the menopausal status as variables of the model gave a better discriminatory ability of AUC= 0.8 and had a good predictive capacity when tested using Hosmer and Lemeshow test (p=.502).
Strengths and Limitations
It is clear that in this study we analysed ovarian masses of a relatively small sample number (29 malignant) this is due to several reasons. First, high rate of refusion of participating due to poor general health. Second, some malignant diseases were disseminated so can not be viewed or analysed on ultrasound image. Third, ovarian cancer probability is higher in older postmenopausal women (>70 years old) which made it difficult to approach these women and ask for their participation in the study. These and the limited duration of recruitment time that was approved by the ethical committee (18 months) as well as data were collected from single centre all participate in not achieving number of sample that was desired.
Conclusion
This study has shown that quantitative texture analysis of B-mode images demonstrates a significance difference between cysts, benign and malignant tissue using GLCM and wavelet features. Therefore, it is possible to conclude that using GLCM and wavelet as a computer aided diagnosis has the potential to differentiate objectively the ovarian lesions. It is expected that this method would ultimately improve the diagnosis of medical imaging in general and ovarian masses in particular.
This study has also compared texture analysis features with other widely used scoring systems to diagnose ovarian cancers such as RMI, PMI and ADNEX model. It was found that GLCM diagnostic performance was superior to RMI and ADNEX. This could aid the diagnostic performance in the case of less experienced sonographers.
From our study, we found that texture analysis had slightly better performance when applied to premenopausal women than other scoring systems such as, RMI, PMI and ADNEX models compared to the performance in postmenopausal women.
Combining the texture fetures in a logistic regression model can increase the performance of diagnosing ovarian masses.
Future Work
To assess the reproducibility of texture analysis features on ultrasound ovarian masses images, a multicentre prospective study is recommended with a larger sample to get more representative results and to confirm the clinical importance of this technique.
Additionally, it might be beneficial to explore the possibility of combining texture analysis with Doppler flow assessment to achieve higher diagnostic performance in discriminating ovarian tissue.
Acknowledgement
We would like to thank Dr. Declan Coleman for his assistance on the technical aspects of texture analysis, and Prof. Len Nokes for his general support.
Details of Ethics Approval
The ethics committee of Dyfed Powys approved this study on 18th July 2013 (Ref. 13/WA/0206).
Funding
This research received no specific grant from any funding agency in the public, commercial, or not-for-profit sectors.
References
- Givens V, Mitchell G, Harraway-Smith C, Reddy A, Maness DL (2009) Diagnosis and management of adnexal masses. American Family Physician 80(8): 815-820.
- Kinkel K, Hricak H, Lu Y, Tsuda K, Filly RA (2000) US characterization of ovarian masses: A meta analysis. Radiology 217(3): 803-811.
- Guerriero S, Ajossa S, Garau N, Piras B, Paoletti AM, et al. (2005) Ultrasonography and color Doppler-based triage for adnexal masses to provide the most appropriate surgical approach. American Journal of Obstetrics and Gynecology 192(2): 401-406.
- Gentry-Maharaj A, Menon U (2012) Screening for ovarian cancer in the general population. Best Pract Res Clin Obstet Gynaecol 26(2): 243256.
- Huchon C, Metzger U, Bats AS, Bensaid C, Chatellier G, et al. (2012) Value of three-dimensional contrast-enhanced power Doppler ultrasound for characterizing adnexal masses. Journal of Obstetrics and Gynaecology Research 38(5): 832-840.
- Palma MD, Gregianin M, Fiduccia P, Evangelista L, Cervino AR, et al. (2012) PET/CT imaging in gynecologic malignancies: A critical overview of its clinical impact and our retrospective single center analysis. Critical Reviews in Oncology/Hematology 83(1): 84-98.
- Iyer VR, Lee SI (2010) MRI, CT, and PET/CT for ovarian cancer detection and adnexal lesion characterization. American Journal of Roentgenology 194(2): 311-321.
- Dutta S, Wang FQ, Fleischer AC, Fishman DA (2010) New frontiers for ovarian cancer risk evaluation: Proteomics and contrast-enhanced ultrasound. American Journal of Roentgenology 194(2): 349-354.
- Hamid B (2011) The Reliability of B-mode transvaginal probe image for the quantitative texture analysis and the dependence of extracted features on region of interest size for ovarian cancer detection. In Department of Medical Physics. Cardiff University, Cardiff.
- Giger ML, Chan HP, Boone J (2008) Anniversary paper: History and status of CAD and quantitative image analysis: The role of Medical Physics and AAPM. Medical Physics 35(12): 5799-5820.
- Coleman DP, Rakebrandt F, Pugh ND, Crawford DC, Woodcock JP (2005) Development and validation of an in vivo analysis tool to identify changes in carotid plaque tissue types in serial 3-D ultrasound scans. Ultrasound in Medicine and Biology 31: 329-335.
- Huang YL, Chen DR, Jiang YR, Kuo SJ, Wu HK, et al. (2008) Ultrasound in Obstetrics and Gynecology. 32(4): 565-572.
- Ramaraj M, Raghavan S (2011) A survey of wavelet techniques and multiresolution analysis for cancer diagnosis. In Computer, Communication and Electrical Technology (ICCCET), 2011 International Conference on 109-114.
- Vince DG, Dixon KJ, Cothren RM, Cornhill JF (2000) Comparison of texture analysis methods for the characterization of coronary plaques in intravascular ultrasound images. Computerized Medical Imaging and Graphics 24(4): 221-229.
- Smutek D, šára R, Sucharda P, Tjahjadi T, švec M (2003) Image texture analysis of sonograms in chronic inflammations of thyroid gland. Ultrasound Med Biol 29(11): 1531-1543.
- Xian GM (2010) An identification method of malignant and benign liver tumors from ultrasonography based on GLCM texture features and fuzzy SVM. Expert Systems with Applications 37: 6737-6741.
- Vicas C, Lupsor M, Socaciu M, Badea R, Nedevschi S (2011) Texture analysis as a noninvasive tool for fibrosis assessment in chronic hepatitis C. influence of expert dependent variability over the performance of texture analysis. In Intelligent Computer Communication and Processing (ICCP), 2011 IEEE International Conference on 205-212.
- Kumar SS, Moni RS, Rajeesh J (2012) Liver tumor diagnosis by gray level and contourlet coefficients texture analysis. In Computing, Electronics and Electrical Technologies (ICCEET), 2012 International Conference on 557-562.
- Castellano G, Bonilha L, Li LM, Cendes F (2004) Texture analysis of medical images. Clinical Radiology 59(12): 1061-1069.
- Jacobs I, Oram D, Fairbanks J, Turner J, Frost C, et al. (1990) A risk of malignancy index incorporating CA 125, ultrasound and menopausal status for the accurate preoperative diagnosis of ovarian cancer. British Journal of Obstetrics and Gynaecology 97(10): 922-929.
- Yazbek J, Aslam N, Tailor A, Hillaby K, Raju KS, et al. (2006) A comparative study of the risk of malignancy index and the ovarian crescent sign for the diagnosis of invasive ovarian cancer. Ultrasound in Obstetrics and Gynecology 28(3): 320-324.
- van den Akker PAJ, Aalders AL, Snijders MPLM, Kluivers KB, Samlal RAK, et al. (2010) Evaluation of the risk of malignancy index in daily clinical management of adnexal masses. Gynecologic Oncology 116(3): 384-388.
- Sinah A, Drews F, Lim K, Pugh N (2016) Retrospective analysis of suspicious pelvic masses using the Pelvic Mass Index (PMI) scoring system from 2007 to 2014. Eur J Obstet Gynecol Reprod Biol 201: 7984.
- Van Calster B, Van Hoorde K, Valentin L, Testa AC, Fischerova D, et al. (2014) Evaluating the risk of ovarian cancer before surgery using the ADNEX model to differentiate between benign, borderline, early and advanced stage invasive, and secondary metastatic tumours: Prospective multicentre diagnostic study. BMJ 349: 5920.
- Vidya KS, Ng EYK, Acharya UR, Chou SM, Tan RS, et al. (2015) Computer- aided diagnosis of Myocardial Infarction using ultrasound images with DWT, GLCM and HOS methods: A comparative study. Computers in Biology and Medicine 62: 86-93.
- Ramos RP, Nascimento MZd, Pereira DC (2012) Texture extraction: An evaluation of ridgelet, wavelet and co-occurrence based methods applied to mammograms. Expert Systems with Applications 39: 11036-11047.
- Singh M, Singh S (2002) Spatial texture analysis: A comparative study. Proceedings - International Conference on Pattern Recognition 16: 676-679.
- Van Nagell JR, DePriest PD (2005) Management of adnexal masses in postmenopausal women. Am J Obstet Gynecol 193(1): 30-35.
- Varras M (2004) Benefits and limitations of ultrasonographic evaluation of uterine adnexal lesions in early detection of ovarian cancer. Clin Exp Obstet Gynecol 31(2): 85-98.
- Diamond J, Anderson NH, Bartels PH, Montironi R, Hamilton PW (2004) The use of morphological characteristics and texture analysis in the identification of tissue composition in prostatic neoplasia. Human Pathology 35(9): 1121-1131.
- Menon U, Gentry-Maharaj A, Hallett R, Ryan A, Burnell M, et al. (2009) Sensitivity and specificity of multimodal and ultrasound screening for ovarian cancer, and stage distribution of detected cancers: results of the prevalence screen of the UK Collaborative Trial of Ovarian Cancer Screening (UKCTOCS). The Lancet Oncology 10(4): 327-340.
- Zimmer Y, Tepper R, Akselrod S (2003) An automatic approach for morphological analysis and malignancy evaluation of ovarian masses using B-scans. Ultrasound Med Biol 29(11): 1561-1570.
- Kaijser J, Sayasneh A, Van hoorde K, Ghaem-maghami S, Bourne T, et al. (2014) Presurgical diagnosis of adnexal tumours using mathematical models and scoring systems: A systematic review and meta-analysis. Hum Reprod Update 20(3): 449-462.
- Materka A (2004) Texture analysis methodologies for magnetic resonance imaging. Dialogues in Clinical Neuroscience 6(2): 243-250.
- Tesar L, Shimizu A, Smutek D, Kobatake H, Nawano S (2008) Medical image analysis of 3D CT images based on extension of Haralick texture features. Computerized Medical Imaging and Graphics 32(6): 513-520.
- Tuceryan M, Jain AK (1998) The Handbook of Pattern Recognition and Computer Vision: World Scientific Publishing Co, Singapore.
- Garra BS, Krasner BH, Horii SC, Ascher S, Mun SK, et al. (1993) Improving the Distinction Between Benign and Malignant Breast Lesions: The Value of Sonographic Texture Analysis. Ultrasonic Imaging 15(4): 267-285.
- Michail G, Karahaliou A, Skiadopoulos S, Kalogeropoulou C, Terzis G, et al. (2007) Texture analysis of perimenopausal and post-menopausal endometrial tissue in grayscale transvaginal ultrasonography. British Journal of Radiology 80(956): 609-616.