"To advance the creation, communication and application of scientific knowledge to benefit society and improve people's lives. The prime mission of juniper publishers is to make continuous efforts in transforming the scientific information from researchers around the world, into easily accessible data by publishing on our website. Our vision is to build an open science platform where everybody has an equal opportunity to seek, share and generate knowledge, empowering researchers and scholars in their daily work."
Juniper Publishers Vision
Latest Articles
Review Article

Beyond the Nephron: Exploring Systemic Implications of Renal Tubular Acidosis
José C Rosario Curcio, Tania Elizabeth Salvador, Alba Vidal Ronchas, Carlos E Díaz Ortiz, Nune Azaryan Dermenjian, Vianka Vanessa Yánez Montalvo, Melanie Dayana Yánez Montalvo, Marcela Garzon, Fátima Melissa Morales Navarro, Elizabeth Alfaro PereiraJOJ Urology & Nephrology
Short Communication

Research Article

Determination of Reproductive Period and Sex Inversion Pagellus bogaraveo (Sparidae) in Syrian Marine Waters
Waad George Sabour* and Mai Mostafa MasriOceanography & Fisheries Open Access Journal
Case Report

Electromagnetic stimulation to improve muscle tone in the abdomen and lower limbs: an objective assessment with Magnetic Resonance Imaging
Marta Codognotto, Alessandra Comito, Ilaria Rossi, Beatrice Marina Pennati, Irene Fusco* and Tiziano ZingoniGlobal Journal of Addiction & Rehabilitation Medicine
Review Article

Research Article

Research Article

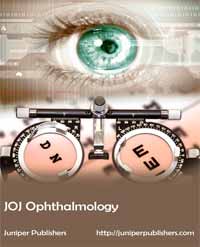
Determinants of the Acceptability of Cataract Surgery in the Community of Sakete, Benin
Abouki Chakiratou Olaïdé Adoukê*, Alpha Bio Amadou, Aïgbé Nestor, Kikissagbe Audiac Towanou, Alamou Soulé and Odoulami LisetteJOJ Ophthalmology
Research Article

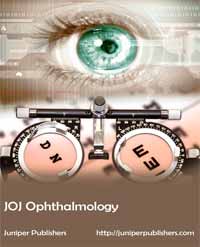