Predicting the Civil Engineering Characteristics through Soft Computing Models
Zahra Keshavarz*
Department of Civil Engineering, Islamic Azad University, Iran
Submission: August 05, 2017; Published: August 22, 2017
*Corresponding author: Zahra Keshavarz Graduate Student, Civil Engineering Department, Islamic Azad University Eslamshahr Branch, Eslamshahr, Iran, Email: zahrakeshavarz.88@gmail.com
How to cite this article: Zahra K. Predicting the Civil Engineering Characteristics through Soft Computing Models. Civil Eng Res J. 2017; 1(3): 555563. DOI: 10.19080/CERJ.2017.01.555563
Abstract
Recently, scientists have used different soft computing models for estimating various civil engineering characteristics, since they are economical, reliable, and time-saving. Among all, Adaptive Neuro-Fuzzy Inference System (ANFIS) and Artificial Neural Network (ANN) have been the most efficient ones, which are used by scientists in multiple occasions. This study is the comprehensive review on capability of ANFIS and ANN models in predicting different civil engineering characteristics.
Keywords: Artificial neural network; Neuro-fuzzy inference system; Fuzzy inference system
Review and Discussion
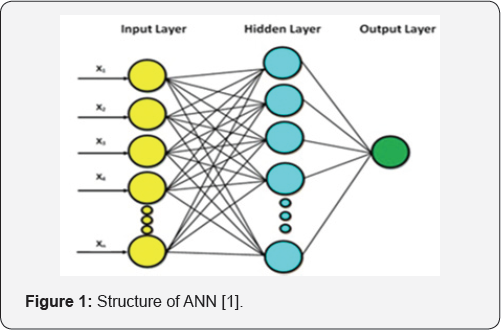

Recently, various data-driven models such as ANFIS and ANN and their hybrids have been used for predicting various civil engineering characteristics. ANN is a data processing system which learns from the examples to get to a specific problem [1]. In addition, ANFIS is a famous hybrid neuro-fuzzy method for modeling nonlinear complex structures which incorporates the adaptive learning algorithm of ANN with the reasoning capability of fuzzy inference system (FIS) [2]. The structures of ANN and ANFIS are shown in Figures 1 & 2, respectively.
Scientists have used the applications of ANN and ANFIS models successfully in various occasions in the field of Civil Engineering. Nazari et al. [3] have used ANFIS effectively in estimating ductile to brittle transition temperature offunctionally graded steel. Prasad et al. [4] have developed ANFIS productively in predicting the air quality and input optimization to be able to save cost and time. Khademi et al. [5] have used ANN and ANFIS models successfully in estimating the 28 days compressive strength of concrete, however, they also concluded that ANN is more capable than ANFIS in such a prediction purposes. Boga et al. 2013 evaluated the mechanical and chloride permeability properties of concrete containing GGBFS and CNI through ANN and ANFIS techniques efficiently [6].
As a result, Adaptive Neuro-Fuzzy Inference System (ANFIS) and Artificial Neural Network (ANN) are found to be reliable in predicting different characteristics in the field of Civil Engineering and are being used by different scientists around the world [1-6]. Using these soft computing models are economical, time-saving, and easy to be used which would be the solution for many projects which these parameters are important in them.
References
- Khademi F, Jamal SM, Deshpande N, Londhe S (2016) Predicting strength of recycled aggregate concrete using artificial neural network, adaptive neuro-fuzzy inference system and multiple linear regression. Intl J Sustble Built Envt 5(2): 355-369.
- Khademi F, Akbari M, Nikoo M (2017) Displacement Determination of Concrete Reinforcement Building using Data-Driven models. International Journal of Sustainable Built Environment, DOI: 10.1016/j.ijsbe.2017.07.002.
- Nazari A, Milani AA, Khalaj G (2012) Modeling ductile to brittle transition temperature of functionally graded steels by ANFIS. Applied Mathematical Modelling 36(8): 3903-3915.
- Prasad K, Gorai AK, Goyal P (2016) Development of ANFIS models for air quality forecasting and input optimization for reducing the computational cost and time. Atmospheric environment 128 (2016): 246-262.
- Khademi F, Akbari M, Jamal SM, Nikoo M (2017) Multiple linear regression, artificial neural network, and fuzzy logic prediction of 28 days compressive strength of concrete. Frontiers of Structural and Civil Engineering 11(1): 90-99.
- Motamedi S, Shamshirband S, Petkovic D, Hashim R (2015) Application of adaptive neuro-fuzzy technique to predict the unconfined compressive strength of PFA-sand-cement mixture. Powder Technology 278: 278-285.